The Quest for Ethical Machine Learning in Computational Neuroscience
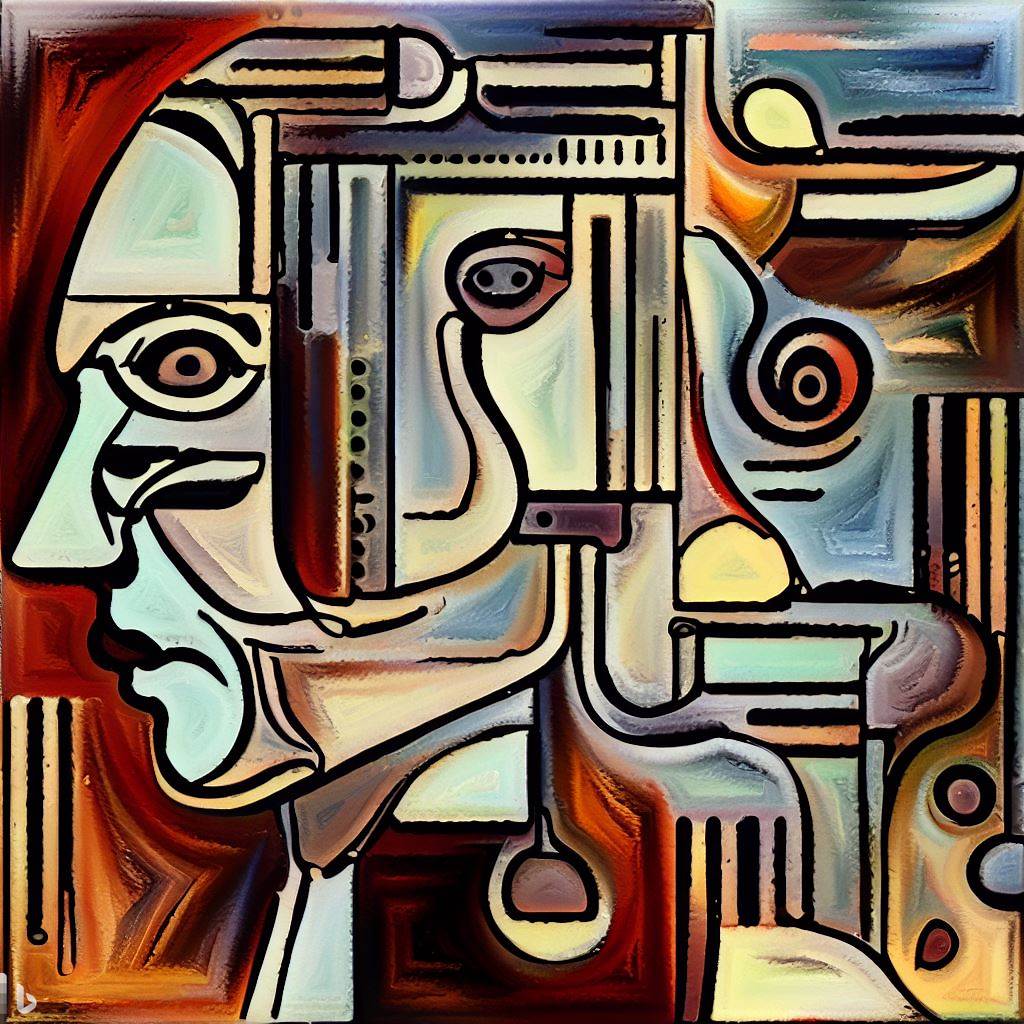
Hey neurons,
This week, I've been delving into the intriguing world of using machine learning in computational psychiatry. For those unfamiliar with the term, Computational Psychiatry is a field that employs computational methods to understand the mechanistic underpinnings of psychiatric disorders. It seeks to model the brain processes related to cognition, emotion, and behaviour, drawing upon principles from neuroscience, psychology, and computer science. Often researchers try to predict the risk of the future event of a mental health episode. When dealing with such a sensitive domain as mental health disorders, it's imperative to tread cautiously. This involves recognizing not just our own inherent biases but also those that might inadvertently seep into our machine-learning algorithms.
Through my exploration, I've pinpointed five critical guidelines for navigating machine learning within computational psychiatry. While this isn't an all-encompassing list, it provides a roadmap for those curious about or involved in the discipline:
- Assemble a Diverse Team: Diversity in thought and background ensures a holistic view, helping spot potential harm that could impact vulnerable groups.
- Conduct Comprehensive Literature Reviews: Delve into past research to identify instances where machine learning has exhibited biases or been unfair. This helps in understanding and preempting pitfalls.
- Continual Monitoring & Evolution: The field of computational psychiatry is ever-evolving. Regularly review and update your system to reflect the latest scientific insights and findings.
- Encourage Transparency & Interpretability: Opt for models that are interpretable, where possible. This allows for a better understanding of how decisions are made, which is crucial in the medical field.
- Seek Patient or Participant Feedback: Engage with those directly impacted by the algorithms — the patients. Their feedback can provide valuable insights into the real-world implications and acceptability of the computational models.
In the realm of computational neuroscience and psychiatry, ethics isn't just a consideration—it's fundamental. So, whether you're a novice or a seasoned expert, keeping these guidelines in mind can ensure the work you do is not just innovative, but also responsible.
👀 Something to watch
Computational and statistical reproducibility in data-intensive Neuroscience - Gaël Varoquaux - I found this lecture really insightful as Dr. Varoquaux touches upon several challenges of using machine learning in computational neuroscience. For example, the challenge of drawing conclusions from large data sets, as the mere presence of data doesn't guarantee scientific insights. He stresses the need for a clear working hypothesis to guide data analysis, pointing out that without this, one might find correlations purely by chance. The current publication system, which favours positive, high-impact results, further complicates matters by introducing biases.
📚 A Must-Read Paper
📚 Paper - A stable and replicable neural signature of lifespan adversity in the adult brain. This recent study employs normative modelling, an emerging technique in imaging neuroscience that helps identify individual variances. Environmental challenges can lead to long-term changes in our brain structure, impacting our risk of psychiatric disorders. The study observed young adults and found that those with more significant brain changes due to past adversities were more prone to future anxiety. This research highlights the lasting brain effects of environmental stressors and the importance of examining individual reactions to better understand mental health.
Happy coding 👩💻!